土壤有机碳时空变异模拟研究取得进展
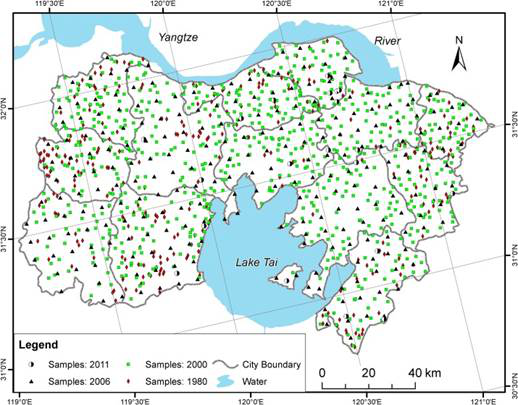
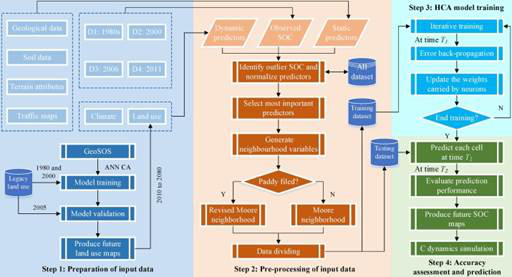
受限于土壤样点的时间维属性,一般的土壤制图只能获得固定时间的“静态”土壤图,描述目前或过去状态的格局。但是,土壤在不同土地利用条件下未来如何演变,在不同的气候变化情景下呈现怎样的时空变化特征?这是土壤时空变化预测的重要主题。
中国科学院南京土壤研究所张甘霖课题组副研究员宋效东以土地利用变化频繁的太湖周边地区为例,深入研究了土壤有机碳含量空间变异的主导因素,提出了基于土壤发生理论的启发式元胞自动机模型,模拟土壤有机碳含量在土地利用与气候变化条件下未来60年内的时空变异特征。有别于常规的元胞自动机模型,该方法不仅能够有效集成影响土壤有机碳含量的静态/动态环境变量,还能够根据土地利用类型(旱地/水田)动态地度量有机碳富集对临近区域有机碳水平迁移的影响范围。鉴于土地利用类型的重要性,根据历史土地利用图层制作了研究区未来60年的土地利用变化图。模拟结果表明:研究区土壤表层有机碳含量在未来60年内随着气温、降雨的升高与城镇化进程的推进将呈现持续上升的趋势。研究提出的预测模型为土壤属性的时空变异模拟提供了新的解决方案与思路。
该研究成果发表在Agriculture, Ecosystems and Environment上。研究得到国家重点研发计划(2017YFA0603002)、科技基础性工作专项(2008FY110600)、国家自然科学基金(41571130051和41771251)的资助。(来源:中国科学院南京土壤研究所)
Heuristic cellular automaton model for simulating soil organic carbon under land use and climate change: A case study in eastern China
Abstract The concentration of soil organic carbon (SOC) is one of the most important soil properties, and its spatio-temporal variability greatly affects the global climate and agroecology. To investigate the effects of land use and climate change on SOC, a heuristic cellular automaton (HCA) model was proposed and applied to a plains area in eastern China with a high population density and rapid urbanization rate. The HCA model was designed to simulate the geographical variation in SOC dynamics over the long term (2080), and lateral carbon (C) migration is represented by revised neighbourhood variables at the macro scale. Three widely used soil mapping techniques were applied for comparison: multiple linear regression (MLR), support vector machine (SVM) and kriging with external drift (KED). The HCA model enhanced the accuracy of the predicted SOC by 15.27% over MLR, 12.31% over SVM and 10.98% over KED. Future land use maps were produced using legacy land use data and artificial neural network-based cellular automata (CA), and the simulation results showed the rapid urbanization of this area, where the percentage of cropland declined by 23.75% and that of village/urban areas increased by 22.90% from 2010 to 2080. The overall SOC concentrations are anticipated to increase by 2080 given the rising mean annual air temperature and mean annual precipitation. Our results also suggested that land use change clearly influenced the change in soil C, with village/urban areas exhibiting higher SOC than cropland. To provide stakeholders with accurate soil information, it is important to understand the comprehensive impacts of land use and climate change on soil evolution; this study illustrates the value of integrating pedogenetic information in soil C simulation models.